Table of Contents
Introduction
The introduction of generative AI into business represents a significant milestone in driving innovation and efficiency. It uses complex algorithms, including deep learning and neural networks, to mimic human creativity and decision-making processes.
Organizations are discovering the value embedded in their datasets as AI applications expand to analyze customer behaviors, optimize processes, and introduce generative AI (GenAI), allowing businesses to unlock new value.
Integrating generative AI into business operations entails not just enhancing existing processes, but instigating a transformational shift that opens new avenues for growth, innovation, and competitive advantage.
Understanding the Basics of Generative AI
Artificial Intelligence is transforming the landscape of technology by enabling machines to understand and create new content autonomously. Below, we delve into the core technologies that power generative AI, explore its various applications, and outline the significant advantages it offers to businesses.
Key Technologies Behind Generative AI

- Machine Learning (ML): At its core, generative AI utilizes ML techniques that allow systems to learn from data patterns and make decisions with minimal human intervention.
- Deep Learning (DL): A subset of machine learning, deep learning uses layered neural networks to analyze various factors of data inputs. These networks mimic human brain functions to a degree, enabling sophisticated problem-solving and creativity.
- Neural Networks: Specifically, generative AI often employs a type of neural network called Generative Adversarial Networks (GANs). These consist of two parts: a generator that creates outputs (like images or text) and a discriminator that evaluates their authenticity.Â
Types of Generative AI Applications

- Text Generation: AI models that can write convincing text, useful for content creation, customer service bots, and even novel writing.
- Image Creation: Tools that generate new images from scratch or modify existing ones, used in design, advertising, and entertainment.
- Data Synthesis: AI that can produce realistic datasets that are invaluable in training other AI systems without the need for real, often sensitive, data.
Advantages of Integrating Generative AI

- Efficiency: Automates and accelerates tasks, allowing human resources to be redirected to more strategic activities.
- Scalability: Can handle increasing workloads or demands without a drop in performance.
- Innovation: Offers the capability to solve problems and generate solutions that are beyond traditional human approaches.
- Personalization: Enables the creation of tailored content or solutions at an individual level, enhancing customer satisfaction and engagement.
Assessing Your Business Readiness

Before a business can harness the power of generative AI, it must first assess its readiness in terms of current technological capabilities and identify potential areas for AI deployment.
Evaluating Current Technological Infrastructure
- Infrastructure Audit: Review the existing IT infrastructure to ensure it can support AI technologies, including sufficient computational power and data storage.
- Technology Stack Assessment: Determine if the current technology stack is compatible with advanced AI solutions or if upgrades are necessary.
Identifying Potential Areas for AI Implementation
- Process Identification: Pinpoint business processes that are repetitive, data-intensive, and relatively standard across the organization as primary candidates for AI integration.
- Needs Analysis: Conduct a needs analysis to identify gaps in capabilities where AI can bring improvements, such as customer service, data analysis, or operational efficiencies.
Setting Clear Objectives

Setting clear, actionable objectives is crucial for the successful integration of generative AI into business processes.
Defining Goals with Generative AI
- Short-term Goals: These might include immediate enhancements, such as reducing the time for data processing or improving customer interaction quality.
- Long-term Goals: Focus on transformative objectives, such as developing new products or services powered by AI or achieving market leadership in AI innovation.
Metrics for Success
- Establish KPIs that are aligned with the overall business strategy
- Common metrics might include increased productivity, reduced operational costs, revenue growth from AI-enhanced products, and customer satisfaction rates.
By understanding the basics of generative AI, assessing readiness, and setting clear goals, businesses can strategically navigate the path to AI transformation, ensuring that they leverage the full potential of this powerful technology to achieve significant competitive advantages.
Developing a Generative AI Strategy
To effectively leverage generative AI, businesses must develop a comprehensive strategy that involves selecting appropriate AI models and deciding whether to build in-house solutions or buy existing technologies.
Choosing the Right AI Models

- Compatibility with Business Needs: Select AI models that align with specific business goals and operational demands.
- Scalability and Adaptability: Ensure the AI model can scale with business growth and adapt to evolving market conditions.
- Accuracy and Reliability: Choose models known for accuracy and reliability in generating outputs, crucial for maintaining trust and operational stability.
Building or Buying AI Solutions
Building In-House
- Pros: Customization to exact business needs, integration with existing systems, and control over updates and security.
- Cons: Requires significant investment in R&D, skilled personnel, and ongoing maintenance.
Buying Off-the-Shelf
- Pros: Faster deployment, often cost-effective in the short term, and support from vendors.
- Cons: Less flexibility in customization, and dependency on external vendors for updates and support.
Decision-Making Factors

- Cost Analysis: Evaluate the total cost of ownership, including development, implementation, maintenance, and any potential downtime costs.
- Technical Expertise: Assess the in-house team’s capability to develop and maintain AI solutions versus the vendor’s expertise.
- Strategic Impact: Consider how the choice between building or buying will affect long-term strategic goals and competitive positioning.
Implementation: Step-by-Step
The implementation of generative AI should be structured in phases to manage risks and optimize outcomes effectively.
Phase 1: Pilot Testing

- Objective Setting: Define clear goals for the pilot test, including expected outcomes and success metrics.
- Scope and Scale: Start with a small, controlled scope to manage risks and gather detailed insights.
- Feedback Mechanisms: Establish channels for collecting feedback from users and other stakeholders involved in the pilot.
Phase 2: Integration
- Technical Integration: Seamlessly integrate AI technology with existing business systems and workflows.
- Staff Training: Provide comprehensive staff training to ensure they are equipped to use and manage the new AI tools effectively.
- Monitoring Systems: Implement monitoring systems to track the performance of AI applications and ensure they are functioning as intended.
Phase 3: Scaling
- Evaluation of Pilot Outcomes: Analyze the results of the pilot tests to identify areas for improvement or necessary adjustments before wider rollout.
- Expansion Strategy: Develop a strategy for scaling the AI solutions across the organization, which may include extending AI capabilities to more business units or geographical areas.
- Ongoing Support and Maintenance: Plan for ongoing maintenance and continuous improvement of AI systems to adapt to new requirements and emerging technologies.
By following this strategic guide to developing and implementing generative AI, businesses can ensure they not only introduce AI effectively but also capitalize on its full potential to drive significant business transformation and competitive advantage.
Navigating Challenges and Risks
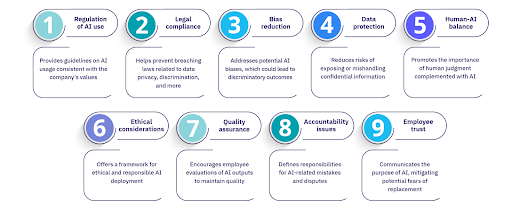
As businesses venture into the realm of generative AI, they encounter several challenges and risks that need to be strategically managed to safeguard their interests and those of their stakeholders.
Ethical Considerations
- Bias and Fairness: AI systems can inadvertently perpetuate biases present in their training data. Implementing measures to detect and mitigate bias is crucial.
- Transparency and Explainability: Businesses should strive to develop AI systems whose decisions can be understood and explained, fostering trust among users.
- Impact on Employment: Addressing the potential displacement of jobs with retraining and reskilling programs for employees affected by AI automation.
Data Security and Privacy
- Compliance with Regulations: Ensuring that AI systems comply with data protection regulations such as the GDPR, which mandates strict guidelines on data usage and consumer privacy.
- Data Integrity: Maintaining the integrity of data used by AI systems, including securing against unauthorized access and ensuring accurate, reliable data inputs.
- Privacy by Design: Incorporating privacy considerations into the development phase of AI projects to protect personal data.
Future Trends and Innovations in Generative AI

The field of generative AI is rapidly evolving, with continuous breakthroughs that promise to further enhance its application and effectiveness.
Latest Developments in AI
- Advanced Neural Architectures: Innovations in neural network designs that allow for more complex and nuanced understanding and generation capabilities.
- Cross-modal AI Systems: Developments in AI models that can integrate and interpret multiple types of data (e.g., text, images, and sounds) to generate richer and more contextually appropriate outputs.
Predictions for Generative AI
- Expansion into New Industries: As AI technology matures, its adoption is expected to spread across more sectors such as healthcare, education, and public services.
- Ethical AI by Default: Increased focus on developing AI systems that are inherently ethical and fair, minimizing risks and enhancing societal acceptance.
- Autonomous Creative Agents: The rise of AI systems capable of producing creative work such as art, literature, and music that may rival human creators.
Conclusion
Generative AI is revolutionizing the business world, offering transformative potential that spans various industries. As businesses look to integrate this advanced technology into their operations, they encounter both vast opportunities and significant challenges.Â
Embracing generative AI is not merely about adopting new technologies but about transforming business models and strategies to thrive in an increasingly digital and automated world. By strategically implementing generative AI, businesses can unlock new possibilities, innovate beyond traditional boundaries, and lead in their respective domains.
Deepak Wadhwani has over 20 years experience in software/wireless technologies. He has worked with Fortune 500 companies including Intuit, ESRI, Qualcomm, Sprint, Verizon, Vodafone, Nortel, Microsoft and Oracle in over 60 countries. Deepak has worked on Internet marketing projects in San Diego, Los Angeles, Orange Country, Denver, Nashville, Kansas City, New York, San Francisco and Huntsville. Deepak has been a founder of technology Startups for one of the first Cityguides, yellow pages online and web based enterprise solutions. He is an internet marketing and technology expert & co-founder for a San Diego Internet marketing company.